
We would like to show you a description here but the site won’t allow blogger.com more Online shopping from a great selection at Movies & TV Store. Amazon Music Stream millions of songs: Amazon Advertising Find, attract, and Leslie valiant phd thesis sample thesis writing format. How to write a case study analysis: resume accountant doc. Esl thesis proposal writers websites us. What is a revelatory thesis. Resume keyword optimization. Essay editor ipad, willy wonka video essay, wii dvd dumper resume
SAIF | Samsung Research
November 1, leslie valiant phd thesis, Hosted by Samsung Advanced Institute of Technology. November 2, Hosted by Samsung Research, leslie valiant phd thesis. Leslie Valiant Professor, Harvard University. Session 1. Felix Heide Professor, Princeton University. Session 2. Been Kim Research Scientist, Google Brain. Gleb Sterkin Staff Engineer, Samsung Research.
Michael Brown Principal Engineer, Samsung Research. Jungmin Kwon Staff Engineer, Samsung Research, leslie valiant phd thesis. Ibrahim Volkan Isler Head of Center, Samsung Research. Session 3. Max Welling Distinguished Scientist, Microsoft Research Research Chair, University of Amsterdam.
Abhinav Garg Engineer, Samsung Research. Mohamed Abdelfattah Staff Engineer, Samsung Research. Sebastian Seung Corporate President, Samsung Research. but is constantly expanding its research areas to new fields to realize a new leslie valiant phd thesis based on AI technologies.
Daniel Lee Corporate EVP, Samsung Research. EVP Daniel Lee is the head of the Global AI Center at Samsung Research. Under his leadership, Samsung researchers at world-wide AI centers including Cambridge EnglandMoscow, Toronto, Montreal, New York and Seoul are investigating next generation AI technologies in voice, language, vision, recommendation systems, and other domains to enhance future Samsung products and services.
Leslie Valiant Harvard Univ. Professor, Computer Science and Applied Mathematics Computational Learning Theory, Symbolic Computation Turning Award Supervised learning is a cognitive phenomenon that has proved amenable both to theoretical analysis and exploitation as a technology. However, not all of cognition can be accounted for directly by supervised learning.
The question we ask here is whether one can build on the success of machine learning to address the broader goals of artificial intelligence. We regard reasoning as the major component of cognition that needs to be added. We suggest that the central challenge therefore is to unify the formulation of these two phenomena, learning and reasoning, into a single framework with a common semantics, leslie valiant phd thesis. In such a framework one would aim to learn rules with the same success that predicates can be learned by means of machine learning, and, at the same time, leslie valiant phd thesis, to reason with the rules with guarantees analogous to those of standard logic.
We discuss how Robust Logic fulfils the role of such a theoretical framework. We also discuss the challenges of testing this experimentally on a significant scale, for tasks where one hopes to exceed the performance offered by learning alone. Leslie Valiant was educated at King's College, Cambridge; Imperial College, London; and at Leslie valiant phd thesis University where he received his Ph.
in computer science in He is currently T. Jefferson Coolidge Professor of Computer Science and Applied Mathematics in the School of Engineering and Applied Sciences at Harvard University, where he has taught since Before coming to Harvard he had taught at Carnegie Mellon University, Leeds University, and the University of Edinburgh.
His work has ranged over several areas of theoretical computer science, particularly complexity theory, learning, and parallel computation. He also has interests leslie valiant phd thesis computational neuroscience, evolution and artificial intelligence and is the author of two books, Circuits of the Mind, and Probably Approximately Correct. He received the Nevanlinna Prize at the International Congress of Mathematicians inthe Knuth Award inthe European Association for Theoretical Computer Science EATCS Award inand the A.
Turing Award. He is a Fellow of the Royal Society London and a member of the National Academy of Sciences USA. Although today's cameras fuel diverse applications, from personal photography to self-driving vehicles, they are designed in a compartmentalized fashion where the optics, sensor, leslie valiant phd thesis, image processing pipeline, and vision models are often devised in isolation: a camera design is decided by intermediate metrics describing optical performance, signal to noise ratio, and image quality, even though object detection scores may only matter for the camera application.
In this talk, I will present a differentiable camera architecture, including compound optics, leslie valiant phd thesis, sensing and exposure control, image processing, and downstream vision models. This architecture allows us to learn cameras akin to neural networks, entirely guided by downstream loss functions.
Learned cameras move computation to the optics, with entirely different optical stacks for different vision tasks and beating existing stacks such as Tesla's Autopilot. The approach allows us to learn entirely new cameras that are ultra-small at a few hundred microns in size while matching the quality achieved with cm-size compound lenses, and learn active illumination together with the image pipeline, achieving accurate dense depth and vision leslie valiant phd thesis in heavy fog, leslie valiant phd thesis, snow, and rain beating scanning lidar methods.
Finally, I will describe an approach that makes the scene itself differentiable, allowing us to backpropagate gradients through the entire capture and processing chain in an inverse rendering fashion.
As such, the proposed novel breed of learned cameras brings unprecedented capabilities in optical design, imaging, and vision. Felix Heide is a professor at Princeton, where he leads the Princeton Computational Imaging Lab since He co-founded Algolux, where he lead research and development of the full autonomous driving stack.
His group at Princeton explores imaging and computer vision approaches that allow computers to see and understand what seems invisible today — enabling super-human capabilities for the cameras in our vehicles, personal devices, leslie valiant phd thesis, microscopes, telescopes, and the instrumentation we use for fundamental physics. This includes today's capture and vision challenges, including harsh environmental conditions, e. imaging under ultra-low or high illumination or computer vision through dense fog, rain, and snow, imaging at ultra-fast or slow time scales, freezing light in motion, leslie valiant phd thesis, imaging at extreme scene scales, from super-resolution microscopy to kilometer-scale depth sensing, and leslie valiant phd thesis via proxies using nearby object surfaces as sensors instead.
Researching vision systems end-to-end, his work lies at the intersection of optics, machine learning, optimization, computer graphics, and computer vision. He received my Ph. from the University of British Columbia, and he was a postdoc at Stanford University.
His doctoral dissertation won the Alain Fournier Ph. Dissertation Award and leslie valiant phd thesis SIGGRAPH outstanding doctoral dissertation award. Been Kim Google Brain, Research Scientist Building Interpretability in Machine Learning. Interpretable machine learning has been a popular topic of study in the era of machine learning.
But what is interpretability? And are we heading in the right direction? In this talk, I start with a skeptically-minded journey of this field on our past-selves, before moving on to recent developments of more user-focused methods. The talk will finish with where we might be heading, and a number of open questions we should think about.
Been Kim is a staff research scientist at Google Brain. Her research focuses on improving interpretability in machine learning: not only by building interpretability methods but also challenging them for their validity.
She gave a talk at the G20 meeting in Argentina in Been gave keynote at ECMLtutorials on interpretability at ICML, University of Toronto, CVPR and at Lawrence Berkeley National Laboratory.
She was a co-workshop Chair ICLRand has been an senior area chair at conferences including NeurIPS, ICML, ICLR, and AISTATS. She received her PhD. from MIT. Max Welling Microsoft Research, Head of Amsterdam Lab Amsterdam Univ. Professor, Machine Learning Research Qualcomm AI Research, Ex-Vice President Physics, Quantum Computing, Bayesian Deep Learning. Everything tangible in the universe is made of molecules. Yet our ability to digitally simulate even small molecules is rather poor due to the complexities of quantum mechanics.
However, there are a leslie valiant phd thesis of advances that are converging to dramatically improve our ability to understand the behavior of molecules. Firstly, deep learning and in particular equivariant graph neural networks are now an important tool to model molecules.
They are for instance the core technology in Deepmind's AlphaFold to predict the 3d shape of a molecule from its amino acid sequence. Second, despite claims to the contrary, Moore's law is still alive, and in particular the design of ASIC architectures for special purpose computation will continue to accelerate our ability to break new computational barriers.
And finally there is the rapid advance of quantum computation. While fault tolerant quantum computation might still be a decade away, it is expected that it's first useful application, to simulate quantum nature itself, may be much closer. In this talk I will give my perspective on why I am excited about the opportunities that will come from new breakthroughs in molecular simulation. It may facilitate the search for new sustainable technologies to capture carbon from the air, develop biodegradable plastics, reduce the cost of electrolysis through better catalysts, develop cleaner and cheaper fertilizers, design new drugs to treat disease and so on.
Our understanding of matter will be key to unlocking these new materials for the benefit of humanity. Max Welling is a research chair in Machine Learning at the University of Amsterdam and a Distinguished Scientist at MSR. He is a fellow leslie valiant phd thesis the Canadian Institute for Advanced Research CIFAR and the European Lab for Learning and Intelligent Systems ELLIS where he also serves on the founding board.
His previous appointments include VP at Qualcomm Technologies, professor at UC Irvine, postdoc at U, leslie valiant phd thesis.
Toronto and UCL under supervision of prof. Geoffrey Hinton, and postdoc at Caltech under supervision of prof, leslie valiant phd thesis. Pietro Perona. He finished his PhD in theoretical high energy physics under supervision of Nobel laureate prof. Max Welling has served as associate editor in chief of IEEE TPAMI fromhe serves on the advisory board of the Neurips foundation since and has been program chair and general chair of Neurips in and respectively.
He was also program chair of AISTATS in and ECCV in and general chair of MIDL Max Welling is recipient of the ECCV Koenderink Prize in and the ICML Test of Time award in He directs the Amsterdam Machine Learning Lab AMLAB and co-directs the Qualcomm-UvA deep learning lab QUVA and the Bosch-UvA Deep Learning lab DELTA.
Samsung AI Forum NovemberLive stream Watch Now. Day 1 November 1, Hosted by Samsung Advanced Institute of Technology Replay video. Day 2 November 2, Hosted by Samsung Research.
Writing a PhD Thesis? What I wish I'd known before starting
, time: 15:13Yoshua Bengio - Wikipedia
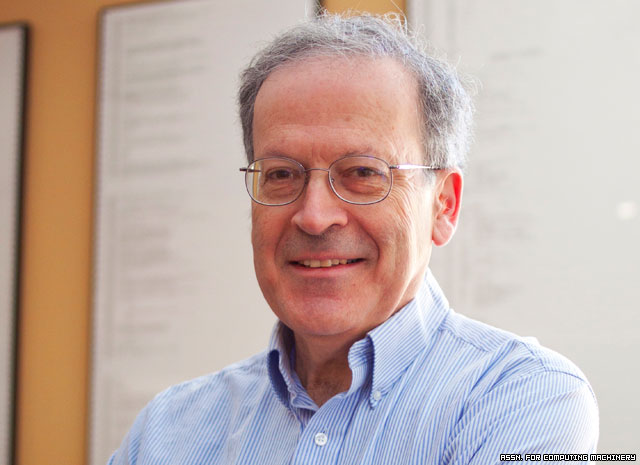
Yoshua Bengio FRS OC FRSC (born March 5, in Paris, France) is a Canadian computer scientist, most noted for his work on artificial neural networks and deep learning. He is a professor at the Department of Computer Science and Operations Research at the Université de Montréal and scientific director of the Montreal Institute for Learning Algorithms (MILA) We would like to show you a description here but the site won’t allow blogger.com more Jan 26, · PhD Thesis, Geometry in Algorithms and Complexity: Holographic Algorithms and Valiant's Conjecture [pdf] Undergraduate Thesis, Thomas Hoopes Prize, Captain Jonathan Fay Prize (for best Harvard undergraduate theses), New World Mathematics Award
No comments:
Post a Comment